AI in Digital Pathology: Hype vs Reality
by Rajendra Singh MD, Co-Founder, PathPresenter
Director of Dermatopathology and Digital Pathology,
Summit Health
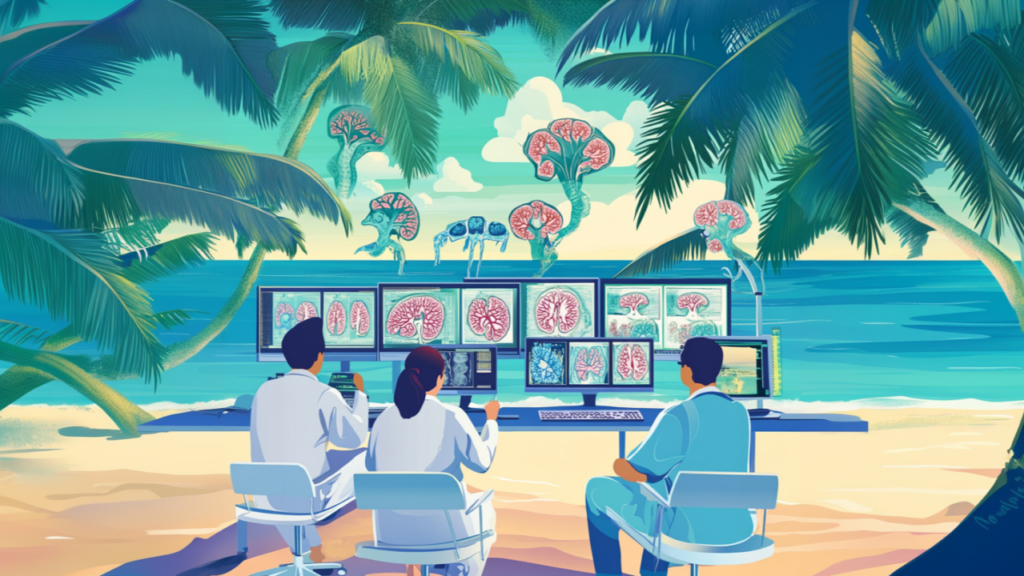
The promise of digital pathology lies in leveraging AI to deliver faster, more accurate, and more efficient diagnoses. Many organizations are eager to adopt these tools, even without the prospect of additional remuneration, due to their potential to alleviate the workload of overburdened pathologists. However, despite the availability of numerous AI models, their real-world implementation remains limited.
As technologists, what steps can we take to ensure that both pathologists and patients benefit from the practical application of these models?
Key Challenges and Solutions
- Data Security and Cloud Integration Most AI models are hosted in cloud environments outside the organization’s DMZ (demilitarized zone), causing reluctance to share sensitive patient data from the IMS (Information Management System) with the model. Proposed Solution: Dockerization of AI modules can enable secure deployment within the organization’s internal infrastructure, addressing data security concerns.
- Integration with IMS Viewers AI-generated outputs—such as heatmaps, annotations, bounding boxes, and textual data—often need to be displayed in the IMS viewer. However, most AI vendors mandate the use of their proprietary viewers, leading to workflow inefficiencies. For instance, a pathologist may need to navigate between multiple viewers during a single session, disrupting the diagnostic process. Proposed Solution: AI results must be seamlessly integrated into the IMS viewer. This approach eliminates the need for multiple viewers and ensures a streamlined, efficient workflow for pathologists. Vendors should prioritize compatibility over exclusivity. This is the approach we’ve taken with PathPresenter; our platform seamlessly integrates AI models from multiple AI vendors and innovators including Paige, IBEX, 4D Path, Mindpeak, and AISencia, giving users the choice of tools with a consistent workflow and UI.
- Validation in Real-World Settings Validation of AI models using an organization’s own data is crucial to ensure reliable performance. Testing in stand alone environments provided by AI vendors often fails to replicate real-world conditions, leading to unmet expectations. Proposed Solution: AI models should be evaluated in a sandbox environment within the organization’s infrastructure with data from the organization. This allows comprehensive testing with real data and ensures the model performs as claimed before deployment.
What challenges do you see in your environment? The real value of digital pathology will come in when AI models not only help in better and faster diagnosis, but can also be deployed for prognostication and prediction of tretament. Such advancements would not only enhance patient outcomes but also justify and incentivize the costs associated with digitization and AI adoption. As the field progresses, we look forward to the emergence of models that address these broader needs, paving the way for improved clinical utility and the integration of compensation frameworks for the use of AI in pathology.
About the Author
Dr. Rajendra Singh is Director of Dermatopathology at Summit Health and co-founder of PathPresenter. He serves as a member of the Digital and Computational Pathology Committee of the CAP, Editorial Board of the WHO for Classification of tumors, 5th Edition and the Board of Digital Pathology Association.